User Item recommendations
User Item recommendations are a simple way to increase sales by making product suggestions to customers based on historical behavior. This can be applied not only to e-commerce but also to music, gaming, events, articles and more. With the Crossing Minds item-based recommendation model, we are capable of extracting item suggestions for each user based on memory and group data. This gives us the capacity to calculate similarities between co-rated items and predict taste outcomes even with insufficient data.
Many companies lack the algorithms in place to prioritize a user’s taste or style in order to make future recommendations after interacting with the product. This is the case, as an example, for large online hotel or home booking sites, which require the user to start at zero each time they search.
These site search engines and filters aid users in completing the singular task of finding a place to stay in a particular city, but are in a deficit when it comes to having algorithms in place to collect user tastes or preferences during their search. In order to accurately recommend hotel rooms or homes that are styled or set-up similarly to places the user has booked before, these companies must find new ways to collect user preferences.
Not only is Crossing Minds capable of categorizing and accurately taste-mapping user preferences, we also utilize cross-domain mapping to determine the recommendation for a user to put them in the right room, in the right city, next to the right restaurants and entertainment. Our taste predictive algorithms are designed to provide fully personalized user experiences across the board.
Definition of Success
For Crossing Minds, the hallmark of a successful recommendation engine is the self-perpetuating, circular improvement to three core tenets:
- Initial learning as user trains the engine on her preferences;
- Increased engagement and usage frequency as user history deepens, recommendations become more granular and system gets smarter;
- Active and loyal user depends on platform for recommendations of all types, ensuring the advancement of the ecosystem
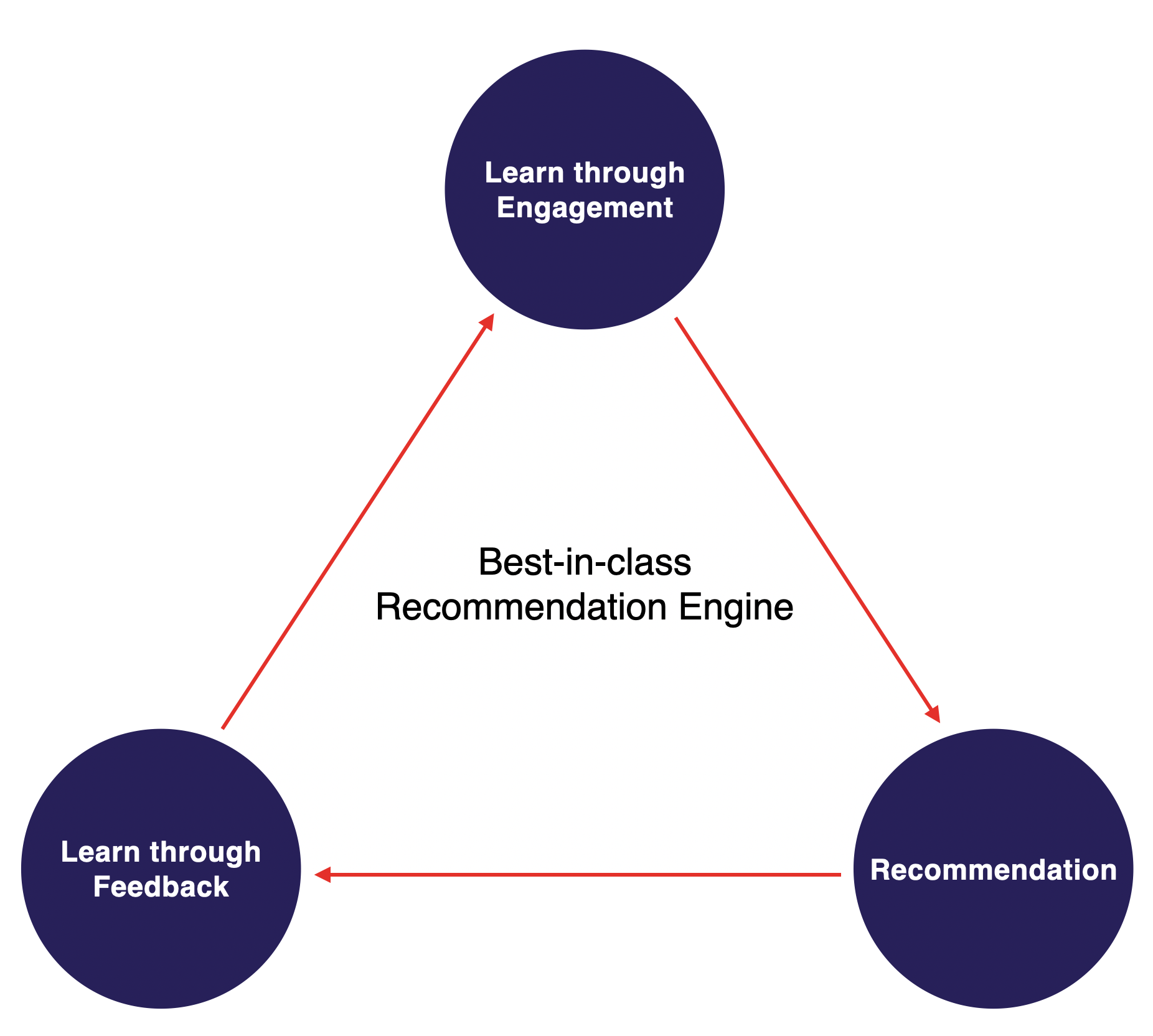
Work with us to improve your customers retention and lead scoring: