Major Publisher
Increase user engagement, increase book sales, and create more personalized recommendations through email
In-store book sales have plummeted as large online companies dominate the market creating a hyper-competitive atmosphere. With a vast multitude of titles available, selling books at rock-bottom prices is no longer the solution for gaining and retaining customers. With a plethora of buying choices, consumers are seeking out more personalized experiences when it comes to their consumption of books.
The challenge
In our work with MP, we strove to create a more customized user experience based on an in-depth study of MP’s user profiles. MP’s decided method of delivering an individualized experience to their users would be through email marketing campaigns.
MP currently sends a bi-weekly personalized email to their 500k+ subscribers containing recommendations of books, unfortunately the recommendation systems needed to convert their customers were not in place. So our goal was to focus on personalization in order to begin optimizing each email for conversions.
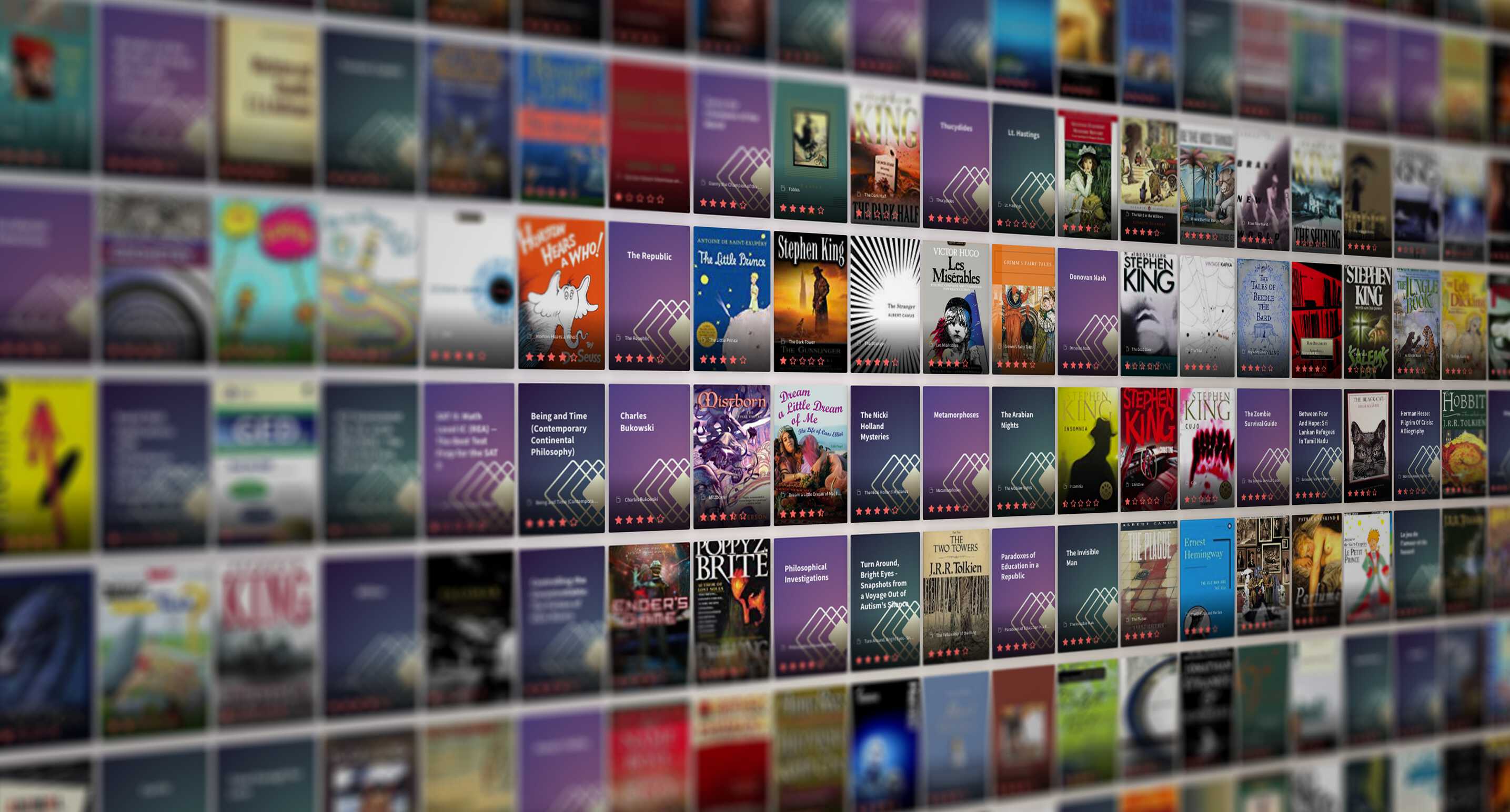
Our approach
Being the core of any supervised recommender system, it is crucial to get accurate insights into the user-item interaction graph. We presented our density analysis of the adjacency matrix, and then further details on the connectivity of the graph to find “information bottlenecks”. Our next step was to pinpoint additional data about the items and on which users it would have the highest impact.
Our dataset took into consideration clicks from users as well as purchases. With our deep learning technology, we were able to aggregate multiple MP datasets to train the algorithm including any new information about the users as well as new items.
This is the point where our software stood out. Because of our knowledge gathered from Hai, and our capability of performing cross-domain recommendations, we were able to greatly increase the knowledge available to the recommender engine. This gave a much deeper insight into ratings of content and user taste maps generated from these profiles.
Results
As a result, MP had more accurate recommendations which led to higher conversions in terms of clicks and sales.
The Crossing Minds solution outperformed the current algorithm used by MP by a significant and reliable margin. Our unique embeddings for the users and items tackled the cold-start problem and accurately provided recommendations to new users. Our efforts also granted MP deeper insight into customer behavior and preferences, which increased overall customer loyalty and purchases. All of this was accomplished while keeping all item data and user data fully de-identified and secure.
Work with us to improve your overall customer loyalty and purchases: